Making Business Decisions During a Pandemic
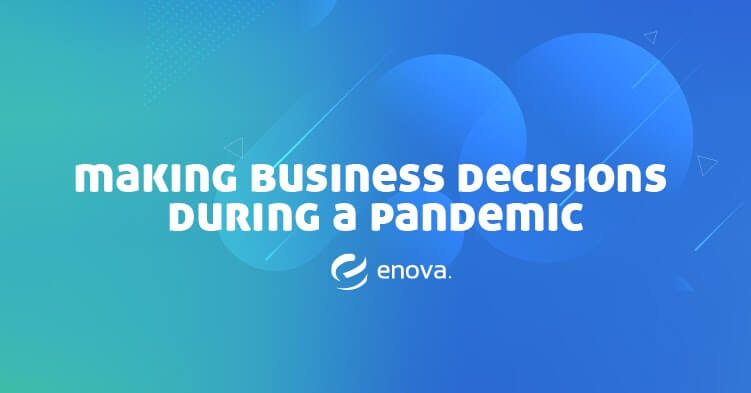
by Mike Ngan, Analytics Senior Manager
At the onset of the pandemic, Enova, as with most businesses, decided to reduce risk immediately. It wasn’t immediately clear at the time how the covid-19 pandemic would progress and how it would impact our customers’ financial situations. One of our immediate goals was to quantify this risk — a task assigned to our newly formed Internal Consultancy team. Even before the pandemic, Enova had a recession monitoring system in place. However, this system was designed to look for signs of a more traditional financial recession, which typically takes about 6 months to form. Given COVID-19 hit much faster than that, we had to design a more specific framework for monitoring a recession caused by this pandemic.
One of the challenges we encountered was that there were no past pandemics in Enova’s history to check the accuracy of a model. We had to make some assumptions from observations thus far in the current pandemic. Even early on, we knew that illness causes business shutdowns, which causes employment loss, which ultimately leads to loan losses. We also observed that outbreaks are regional. From these assumptions, we knew we could model future economic stability expectations and tune our lending strategies around this. Using an aggregate of metrics, such as Google trends, mobility data, hospital occupancy rates, and internal payment metrics, we assigned a 5 point scale to identify where each state was in the pandemic lifecycle. The expectation is that when a state is getting worse, the customer’s future economic situation will be volatile and we should proceed cautiously. If a state is getting better, their economic situation will be stable and we could take more risks.
Based on this metric, a strategy is proposed at each of the 5 points. Although we knew that we wanted to return to normalcy in order of descending reward per unit risk, it’s not clear how much risk was too much. In economics 101 terms, although we were relatively certain that we were still able to rank order customers’ credit quality, we were not clear where marginal cost equals marginal revenue. Thus, the plan to return to lending is through a series of rapid experiments to find the breakeven points. This strategy was modeled after how medicine conducts clinical trials. In states where the framework indicated the economy was still degrading, we would only commit to small dosage, small population tests. In states where the framework indicates the economy was improving, we will commit to larger scale, higher dosage tests.
Over the past few months, the pandemic has evolved at a rapid pace; as such, we need to continuously improve the model to account for these changes. For example, as the summer progressed, governors were less concerned with case growth and more concerned with hospitalization rates to dictate policies that govern businesses. Also, federal reserve intervention disconnected employment to customer economic stability. On the execution side, we also learned that weekly back and forth on lending criteria was unreasonable; so we added autoregressive terms to dampen oscillations. Most importantly, continuous testing has changed what our actions are even if there are no macroeconomic changes.
Our goal was to build an indicator to roughly tell Enova what the regional lending environment was like as we navigate through this pandemic. Over many weeks of iterations and tests, we have made many improvements which continue to give us confidence as to where the best place to allocate our resources are. We’re looking forward to the time when we’re able to return to our traditional methods of making lending decisions, but this framework has allowed us to ensure we’re making smart decisions as a company, during an uncertain time.
Want to be part of the team? Apply here.